Deploying a service with conversation AI can seem daunting, but NVIDIA now has tools to make this process easier, including Neural Modules (NeMo for short) and a new technology called NVIDIA Riva. To save time, pretrained ASR models, training scripts, and performance results are also available on the NGC software hub.
NVIDIA NeMo is a toolkit based on PyTorch created for developing AI applications for Conversational AI. Through modular Deep Neural Networks development, NeMo enables fast experimentation by connecting modules, mixing and matching components. NeMo modules typically represent data layers, encoders, decoders, language models, loss functions, or methods of combining activations. NeMo makes it easy to compose complex neural network architectures and systems using reusable components for each of ASR, NLP and TTS. Additionally, with NVIDIA GPU Cloud (NGC), you can find NeMo resources for conversational AI such as pre-trained models, scripts for training or evaluation, and NeMo end-to-end applications that allow developers to experiment with different algorithms and perform transfer learning using their own datasets.
To facilitate the implementation and domain adaptation of the complete ASR pipeline, NVIDIA created the Domain Specific – NeMo ASR Application. This application is developed using NeMo and lets you train or fine-tune pre-trained (acoustic and language) ASR models with your own data. This gives you the ability to progressively create better performing ASR models specifically built for your data.
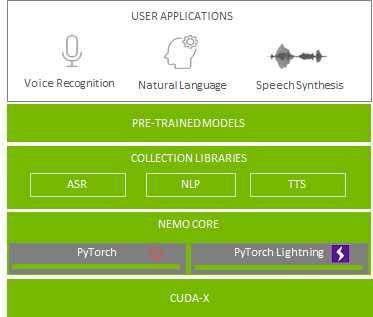
NVIDIA Riva is an application framework that provides several pipelines for accomplishing conversational AI tasks.
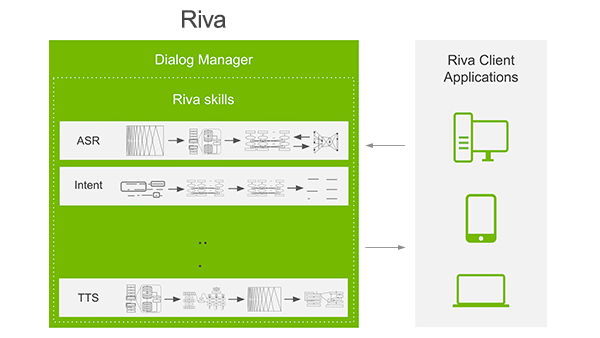
NVIDIA GPU-Accelerated, End-to-End Data Science
The NVIDIA RAPIDS™ suite of open-source software libraries, built on CUDA, gives you the ability to execute end-to-end data science and analytics pipelines entirely on GPUs, while still using familiar interfaces like Pandas and Scikit-Learn APIs.
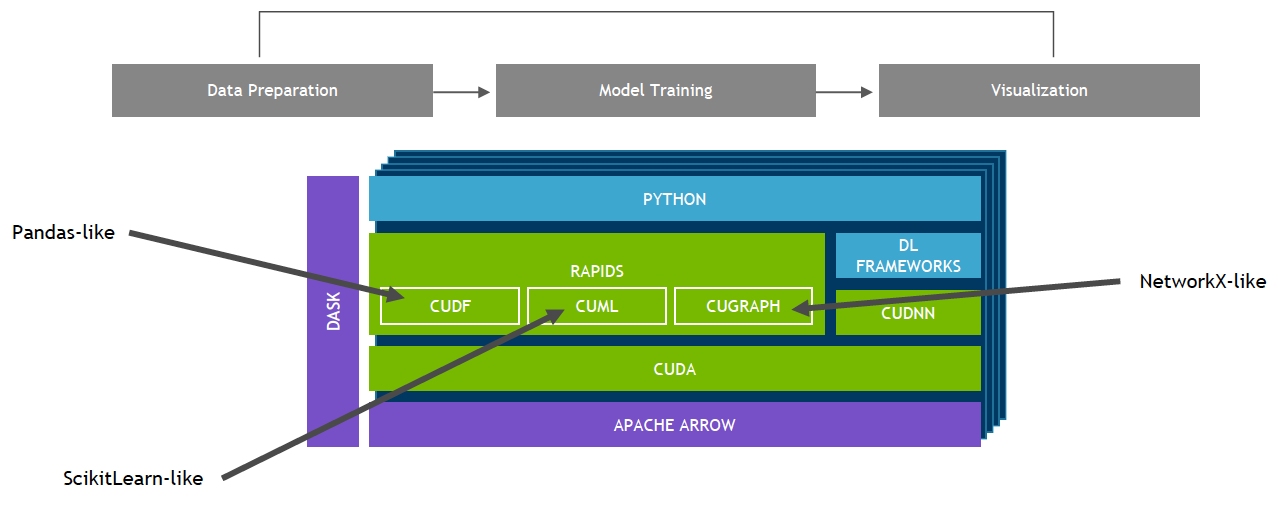
NVIDIA GPU-Accelerated Deep Learning Frameworks
GPU-accelerated deep learning frameworks offer flexibility to design and train custom deep neural networks and provide interfaces to commonly used programming languages such as Python and C/C++. Widely used deep learning frameworks such as MXNet, PyTorch, TensorFlow, and others rely on NVIDIA GPU-accelerated libraries to deliver high-performance, multi-GPU accelerated training.
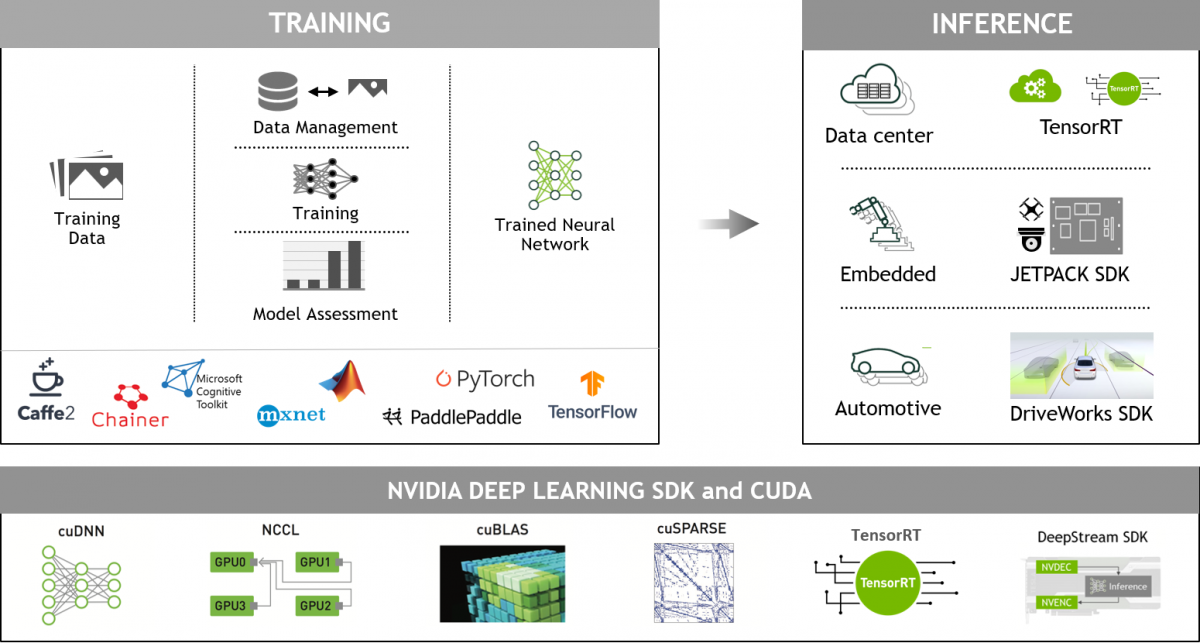