Deep learning uses multi-layered artificial neural networks (ANNs), which are networks composed of several "hidden layers" of nodes between the input and output.
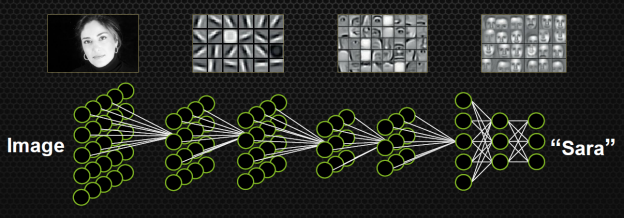
An artificial neural network transforms input data by applying a nonlinear function to a weighted sum of the inputs. The transformation is known as a neural layer and the function is referred to as a neural unit.
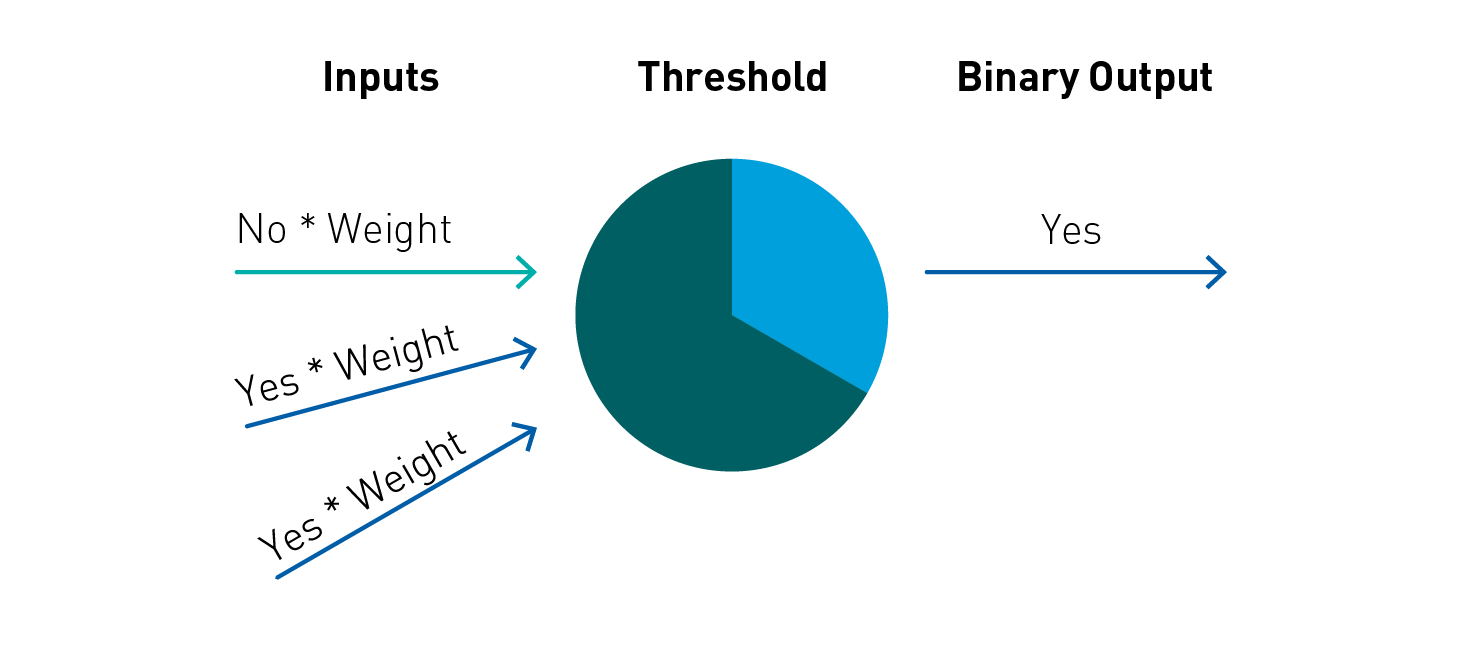
The intermediate outputs of one layer, called features, are used as the input into the next layer. The neural network learns multiple layers of nonlinear features (like edges and shapes) through repeated transformations, which it then combines in a final layer to create a prediction (of more complex objects).
With backpropagation inside of a process called gradient descent, the errors are sent back through the network again and the weights are adjusted, improving the model. The neural net learns by varying the weights or parameters of a network so as to minimize the difference between the predictions of the neural network and the desired values. This process is repeated thousands of times, adjusting a model's weights in response to the error it produces, until the error can't be reduced anymore. This phase where the artificial neural network learns from the data is called training. During this process, the layers learn the optimal features for the model, which has the advantage that features do not need to be predetermined.
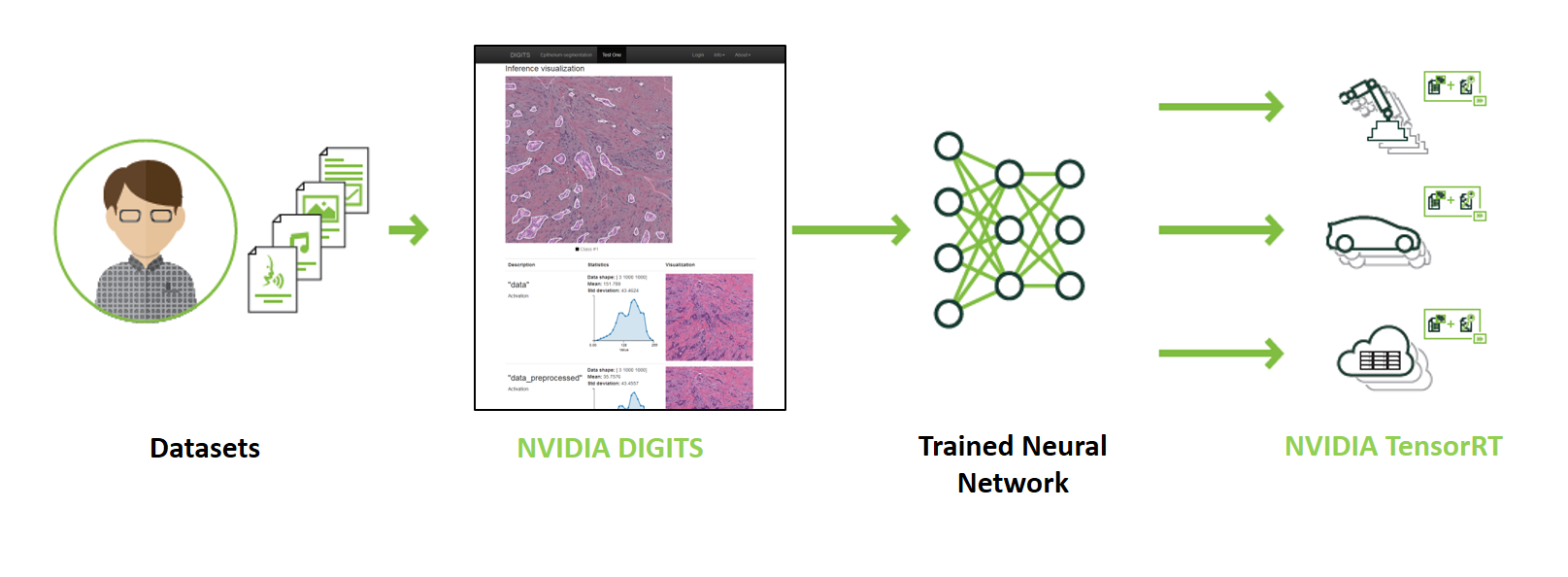
GPUs: Key to Deep Learning
Architecturally, the CPU is composed of just a few cores with lots of cache memory that can handle a few software threads at a time. In contrast, a GPU is composed of hundreds of cores that can handle thousands of threads simultaneously.
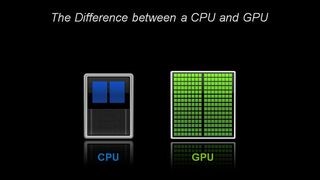
State-of-the-art deep learning neural networks can have from millions to well over one billion parameters to adjust using backpropagation. They also require a large amount of training data to achieve high accuracy, meaning hundreds of thousands to millions of input samples will have to be run through both a forward and backward pass. Because neural nets are created from large numbers of identical neurons, they’re highly parallel by nature. This parallelism maps naturally to GPUs, providing a significant computation speedup over CPU-only training and making them the platform of choice for training large, complex neural network-based systems. The parallel nature of inference operations also lend themselves well for execution on GPUs.